Wow, crazy to think that this was almost a year ago that I was working on this stuff. (likely nothing to do with the job cuts that were announced in April 2021 at my job for which there are ongoing strikes to resolve!)
I may be bumping some older threads as I come to my senses, so do pardon some of my “oldschool” questions and such.
One of the (many) things I want to revisit is this idea of boiling down a corpus-specific(*) descriptor soup which will be then dimensionally reduced down to a 2d/3d plot, to hopefully then trigger using something like this:
Likely using the RGB LEDs to display a quantized UMAP projection:
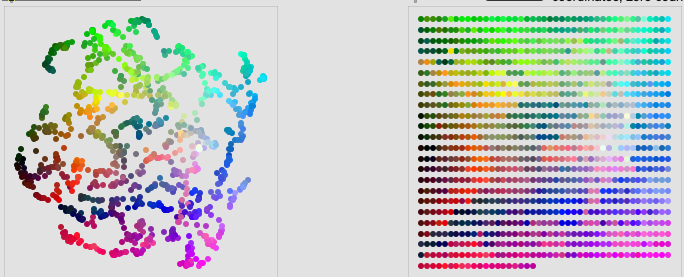
At the time of this original thread we didn’t yet have fluid.grid~
which makes some of the translation stuff simple/trivial now, which is great, but I’m not up to date on whether or not the salience stuff was built into fluid.pca~
and/or if other new additions were added to facilitate that process.
So as a bit of a reminder to myself, and as context, I’ll outline what I’m aiming to do:
- take a corpus of samples (sliced “one shot” samples)
- analyze via the “grab all descriptors and stats” approach
- cook some of the data (loudness-weighted spectral moments, pitch-weighted confidence, etc…)
- use PCA to figure out the most salient components (say 90% coverage or whatever)
- use UMAP to reduce that down to a 2d/3d projection
- quantize the projection to a grid using
fluid.grid~
- potentially apply some perceptual ordering/scaling to the results(?)
- use a kdtree to match the nearest point using XY(Z?) data from the controller
- potentially also retrieve loudness/melband data for loudness/spectral compensation(?)
Following that, here are the more concrete-ish questions I have.
(I know that “it depends” is the answer to everything, but just trying to work out best practice, and a generalizable approach that I can apply, before tweaking in detail)
Now, I remember a lot of lively discussion about normalizing, standardization, robust scaling, etc… I don’t know where that needs to happen in this process. Some things like loudness-weighted spectral moments, and confidence-weighted pitch would definitely go before PCA, but my gut tells me all of it should happen before then. That would potentially require a massive processing chain to treat each statistic of each descriptor differently. Similarly, I guess the output of UMAP may need to be normalized as well before being quantized. Is that right?
Is there a newer or streamlined way to do long processing chains? (e.g. getting loudness, pitch, mfccs, spectral shape, etc… along with all their descriptors into a single flattened buffer) Even just typing this out I’m having flashbacks of spending 2h+ trying to create a single processing chain that takes into consideration the weighting/scaling/etc…
Is there a way of getting the ‘scree’ plot natively now? In the PCA/SVM thread (with @tedmoore and @weefuzzy’s help) there’s some hacky/janky jitter code that gives some results, but it’s not very elegant or robust.
Once you have the salience info, is there a newer or streamlined way to pluck out the desired statistics/descriptors post PCA and pre UMAP? I know that C++ versions of fluid.bufselect~
(and related objects) were created, but as far as I can tell it still requires a complex mesh of counting indices/channels and praying you have the right stuff in the flattened buffer in the end.
Has anything changed on the kdtree (rtree?) front? Like, is it possible to adjust queries and/or have columns that are retrievable but not searchable (like pulling up loudness/melband data, but not using those for the actual query). Before, I think I had a parallel coll
, which is fine, but wondering if there’s a native approach for this “metadata”.
This last one is a bit more nebulous/philosophical, but as useful as it would be to map a bespoke UMAP projection onto that ERAE interface, I really really don’t like the idea of having to “learn” each mapping from scratch. I’d like to have some kind of indication or permanent correlation on the surface and the controller. Also considering that the surface is velocity sensitive, I’d like that to respond to the playing as well (so the louder I hit the surface, the louder that particular sample plays), hence not being sure if I should go with a 2d or 3d projection.
Ages ago I asked about somehow reorienting or re-remapping the UMAP projection using some perceptual descriptors (say centroid or loudness or pitch), without doing a CataRT-like, straight up, 2d plot. Or perhaps using color as a corollary dimension (red always = “bright” etc…).
Maybe some kind of crude clustering after the fact where nodes are created where top right is “bright and pitchy” and bottom left is “dark and noisy” and then cluster the UMAP to that or something?
So yeah, a more ramble-y question this last one, but the basic idea would be to best use the performance surface while not needing to learn each mapping individually.
*I have a more general desire to have a generalizable descriptor soup for matching across input and corpora, but for this purpose there’s no incoming audio to match